Data Science Advice for Interviewees, Master’s Students and Career Changers
In Conversation with Michael Ng, the Data Analytics Manager at Agilent
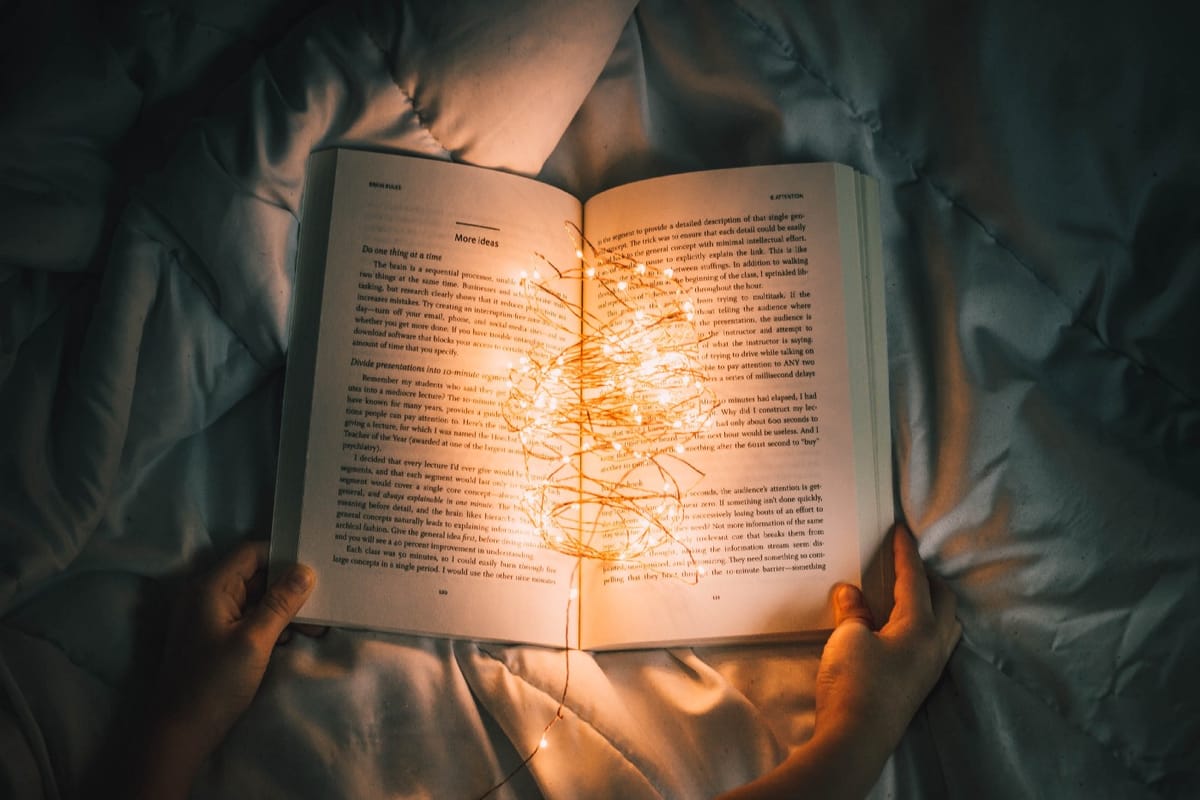
I recently stumbled across Symbolic Connection, a data podcast ran by experienced data practitioners, Thu Ya Kyaw and Koo Ping Shung. This podcast caught my attention as the guests are mainly from Singapore, one of the burgeoning tech hub of South-East Asia (it was also from where I am based.)
As a data analyst in a tech company, I am always curious about the work of analysts in other tech firms. So I listened to the podcast featuring Michael Ng from Agilent.
In this podcast, Michael shared about how his role as a data analyst manager at Agilent, advice for masters students and tips for data interviewees.
Background
Michael is from Agilent technologies, which make life science instruments and consumables for research labs. At Agilent, his team provides BI and data science solutions. Before that, he completed a Masters in IT in Business Analytics and a Bachelors in Marketing and Operations Management at the Singapore Management University.
Agilent delivers complete scientific solutions, helping customers achieve superior outcomes in their labs, clinics, business and the world they seek to improve.
Let’s get to the interview!
What is your day-to-day as a data analytics manager like?
There are three broad responsibilities that Michael handles.
- Producing business intelligence solutions to stakeholders
- Analyze data that can help with the global supply chain at Agilent.
- Find opportunities to grow the company with Industry 4.0 by identifying problems and building proof-of-concepts.
What skills did you find valuable?
To Michael, the valuable skills that he picked are able
- Ability to communicate simply and succinctly. Stakeholders appreciate succinctness. In a deck, it usually suffices to cover three components — what is the situation, what does the data say and what is the solution.
- Resourcefulness. Tools are ever-changing and interchangeable. Therefore it is important to have a breadth of skills and be able to pick up new tools.
- Statistical inferencing or analytical storytelling. For instance, being able to process data and obtain insights from a trend chart.
What do you look out for in an interviewee for data analyst or data scientist?
Interestingly, Michael highlights the importance of good attitude and soft skills over technical skills. In fact, here’s what he looks out for
- A good attitude. Attitude is important because it is immutable, and determines whether this person will work well with the hiring manager or the team.
- Demonstrated ability to solve problems and tell stories. In interviews, Michael looks out for hints that shows how the candidate approach problems and resolve roadblocks.
- Domain expertise. For Agilent (a supply chain company), domain expertise in supply chain earns a candidate credibility.
- Ability to interact with stakeholders.
- Ability to tackle data problems in a short time frame for analysts. This is because analysts are at times expected to produce analysis with a quick turnaround time.
- The interviewee needs to be aware of whether that job is what you want and whether you can get good exposure for the job and can work well with the manager.
Agilent, like many other companies, has technical screening rounds, which consist of three problems.
When asked about the preferred education background of the candidate, Michael mentions that a Master’s or a PhD are not necessary. In fact, a candidate need not have a computer science degree to pass the resume screening, as long as they demonstrated some data work (good news for non-CS majors out there like myself!)
How did you select your Master’s program?
In considering a Master’s programmes, Michael made his decision by breaking down his decision criteria into (at least) four parts.
- Local vs overseas. Michael chose a local degree (in Singapore) as it allows him to build a network locally.
- Part-time vs full-time. As data jobs are inherently demanding, he opted for a full-time class to focus on his learning.
- Relevancy of the degree to his goal. He decided that SMU’s Masters in IT programme was application- instead of theory-driven and was more suited to his goal.
- The faculty member. The value of a Masters program lies in the wisdom of the faculty members and the questions that they could answer.
Any advice for Masters students?
When asked about his time in SMU, Michael reflected on his Master’s studies and gave two advice.
- Reading up. Going to class without doing homework is counterproductive.
- Build unique passion projects in your assignment. Michael advises against building run-of-the-mill projects because it is easy to find similar projects, which is an incentive to simply copy someone else’s code. An example of a unique passion project is done up by his friend Darren, who predicted the value of new Magic the Gathering cards using Natural Langauge Processing (NLP) and whether they will be weakened in future iterations with high accuracy.
Any advice for interviewees trying to pivot into data science?
As an interviewee, try to avoid companies that
- are unsure of their reasons for hiring data analysts and scientists.
- expect results within an unreasonable timeline
Instead, look for companies that
- understand that data science is a journey and might not produce results instantaneously
- carefully consider your background and fit in the company
- engage with you intellectually during the interviews. For instance, one of Michael’s best interview experience involved a discussion on alternative approaches in his projects.
The interviewee should also get to ask or know the following before signing up for a role —
- what resources do I have?
- what deliverables are expected?
- how much time do I have?
Any advice for those considering a career change into data?
- Build up a data portfolio. Michael himself did a project analyzing Singapore parliament discussions.
- Pick up data visualization skills and be able to communicate via visualizations effectively. Michael enthused on how a good visualization should have a low ink-to-data ratio.
- Pick up and practice business storytelling skills. One possible framework is McKinsey’s Scenario, Complication and Resolution Framework.
- Improve your business writing skills. A book that Michael recommends is the Elements of Style by Strunk and White.
Conclusion
Throughout the interview, Michael spoke about the importance of effective communication as a stakeholder. Indeed, as the bridge between the data team and the business stakeholders, data analysts are the gatekeeper of insights and it is our duty to communicate these insights carefully and effectively.
If you’d like to keep in touch with Michael, please feel free to connect with him on his LinkedIn.
Acknowledgement
Special thanks to Michael, Koo and Thu Ya for their permission to publish this article.